In an interview with Zebra Technologies, Rudolf Schambeck, Senior Manager, Machine Vision, shares his expert views on artificial intelligence and its uses in the world of image processing.
Rudolf outlines the differences between artificial intelligence, deep learning and machine learning, explains how AI can benefit Optical Character Recognition (OCR), and reveals how image processing technology can relieve burdens on employees.
Q: Artificial intelligence, deep learning and machine learning are often used interchangeably, but what are the key differences?
Artificial intelligence (AI) is an umbrella term to describe various techniques used to give machines human-like intelligence. There is no single universally agreed definition of what AI means. AI can include machine learning, neural networks, computer vision, deep learning, natural language processing, artificial general intelligence, artificial super intelligence and more. Fifty four percent of manufacturers in Europe (61% globally) expect AI to drive growth by 2029, says Zebra’s 2024 Manufacturing Vision Study.
Machine learning is a subset of AI and refers to the “learning” of AI models that make predictions and classifications. This is achieved via supervised learning, where algorithms learn patterns from existing data and the inputs, outputs and feedback is supervised, or unsupervised learning, where algorithms discover patterns in data without explicit programming by leveraging neural networks. Semi-supervised methods of machine learning involve a mix of labelled and unlabelled data.
Deep learning is a sub-area of machine learning that utilises deep, artificial neural networks, such as convolutional neural networks. These networks of many hidden layers of connected nodes mimic the neural structures of the human brain, and can approximate very complex functions to analyse images, speech or videos, for example. Deep learning is currently one of the most advanced areas of machine learning.
Q: Artificial intelligence in image processing is just one of many areas of application for this technology. For which tasks is it primarily used here?
The benefits of machine vision are evident in industries that place high demands on safety, quality, compliance and efficiency at high speed, such as the automotive, food and beverage, pharmaceutical and electronics industries. In these industries we see machine vision used for quality or end-of-line inspections, traceability of parts along the supply chain, measurements, presence or absence checks, metrology and porosity testing.
Carmakers and electric battery manufacturers are leveraging easier to use deep learning machine vision to inspect the uniformity of surface coating, detect defects in cells, read barcodes and serial numbers, ensure the consistent positioning and application of adhesives and thermal beads, and carry out quality assembly of battery packs with vision-guided robotics.
Deep learning-based machine vision software is an excellent solution for inspection of natural materials with wide natural variations such as textiles, classification, conformal coating inspection, segmentation, feature and anomaly detection. The right combination of hardware and software using deep learning can improve machine vision applications, enable more complex use cases and evolve the role of engineers to think and act more like data and AI specialists.
Nearly three-quarters of manufacturing leaders (71% in Europe, 73% globally) plan to reskill labour to enhance data and technology usage skills, according to Zebra’s study. New cameras, 3D sensors and machine vision platforms with deep learning are capable of overcoming long-term challenges that traditional machine vision systems are unable to tackle.
Q: What advantages does artificial intelligence bring to Optical Character Recognition (OCR)?
Some machine vision solutions come ready out of the box as low/no code solutions that require no prior machine vision knowledge. One example is optical character recognition (OCR) based on deep learning.
Getting OCR inspection right can be challenging. A variety of factors including stylised fonts, blurred, distorted or obscured characters, reflective surfaces, changing lighting environments, and complex, non-uniform backgrounds can make it impossible to achieve stable results using traditional OCR techniques.
Deep learning-based OCR can come with a ready-to-use neural network that is pre-trained using thousands of different image samples. It can deliver high accuracy straight out of the box, even when dealing with very difficult cases. Users can create robust OCR applications in just a few simple steps— without the need for machine vision expertise.
Using an intuitive interface makes set-up easy. Such solutions are also flexible, as they can be deployed on desktop PCs, Android handheld devices, and smart cameras. These advanced deep learning machine vision capabilities are also being combined with 3D scanning for advanced data capture and analysis.
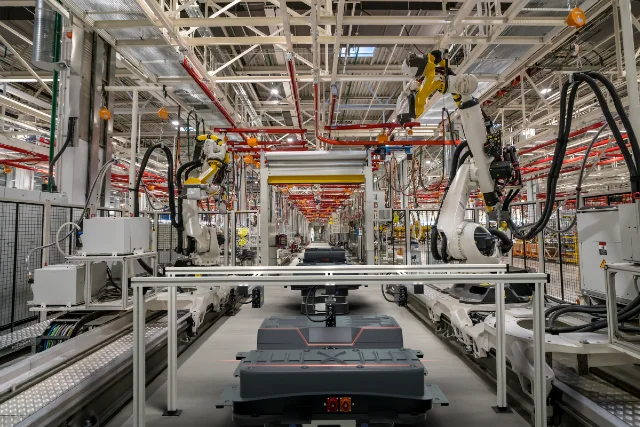
Q: What requirements must be met for a user to be able to use AI for their OCR task? (Which software, which hardware, possibly certain applications?)
An industrial camera designed for visual inspection connected to deep learning-based OCR software is needed, but these solutions are low/no code, fast to set up and require little previous expertise in machine vision, so training staff to use these tools is faster and easier.
A single, standardised platform could be used to set up, deploy and run industrial scanners and machine vision smart cameras. A camera with OCR software could be attached to a robotic arm so the camera can move along and around materials, objects and containers to read codes and serial numbers. An example of how simple it is to set up and use can be seen here, showing how deep learning OCR can quickly and easily read the serial number on an oil filter.
Q: For which applications is it intended? What advantages does it offer there compared to other software with and without AI?
OCR is suitable for a wide range of applications in which letters and numbers on a range of materials need to be read.
The characters might be on metallic, matt, or curved surfaces, engraved, italicised, or embossed and in a range of lighting environments, which makes reading these characters a challenge which standard OCR struggles to handle. Some industry use cases include:
- Automated parcel capture: OCR can read address labels or consignment notes in order to automatically allocate and sort parcels.
- Parts recording and quantity determination: OCR can be used to automatically record parts lists, delivery notes or invoices, e.g. to register goods arrivals.
- Quality inspection: Deep Learning OCR can read production data, batch numbers or expiry dates on packaging and thus support quality assurance.
- Quality control: OCR can recognise production batches, serial numbers, expiry dates etc. on products. This allows traceability and quality assurance to be automated.
- Automated warehouse management: labels, pallet codes and locations in the warehouse can be captured using OCR to document stock levels and stock movements
- Production monitoring: Production figures, throughput times and reject rates etc. can be read automatically from screens or displays.
- Error analysis: OCR also supports the analysis of error photos or log files in order to resolve problems in production lines more quickly
- Tool management: OCR recognises numbers and IDs on tools, devices or machines to monitor their whereabouts or maintenance cycles
Q: Everyone is talking about the shortage of skilled labour. To what extent can modern image processing help to relieve the burden on existing employees?
Six in 10 manufacturing leaders surveyed for Zebra’s 2024 Manufacturing Vision Study rank ongoing development (61% in Europe, 65% globally), retraining/upskilling (66% in Europe, 65% globally), and career path development (63% in Europe, 62% globally) to attract future talent as high priorities for their organisations.
Greater automation, especially in visual inspection using machine vision, can lead to greater accuracy, speed, compliance and safety, so engineers on the production line can delegate inspection tasks to machine vision. It means engineer time is available for other valuable, complex and problem-solving tasks.
Labour recruitment challenges could prove to be another driving factor leading to greater use of machine vision to maintain operations and support the current workforce, as well as acting as a way to attract new talent by demonstrating that employees are equipped with the latest tools to do their best work.
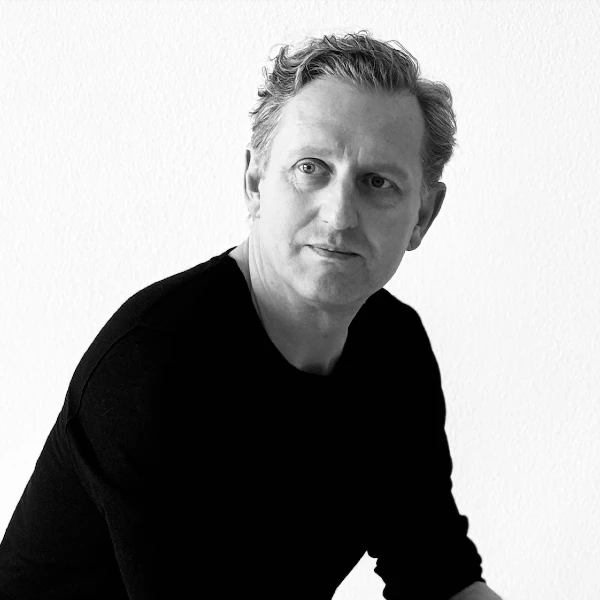
Rudolf Schambeck is the Senior Channel and Market Development Manager, Machine Vision, Germany with Zebra Technologies where he has worked since 2021. Prior to joining Zebra, he spent five years at machine vision company, Cognex in sales engineer, business development, and account manager roles with a focus on B2B and automotive. Prior to Cognex, Rudolf worked for Intercontec Produkt GmbH and Irlbacher Blickpunkt Glas GmbH in B2B and OEM sales engineer, account manager, and sales roles.